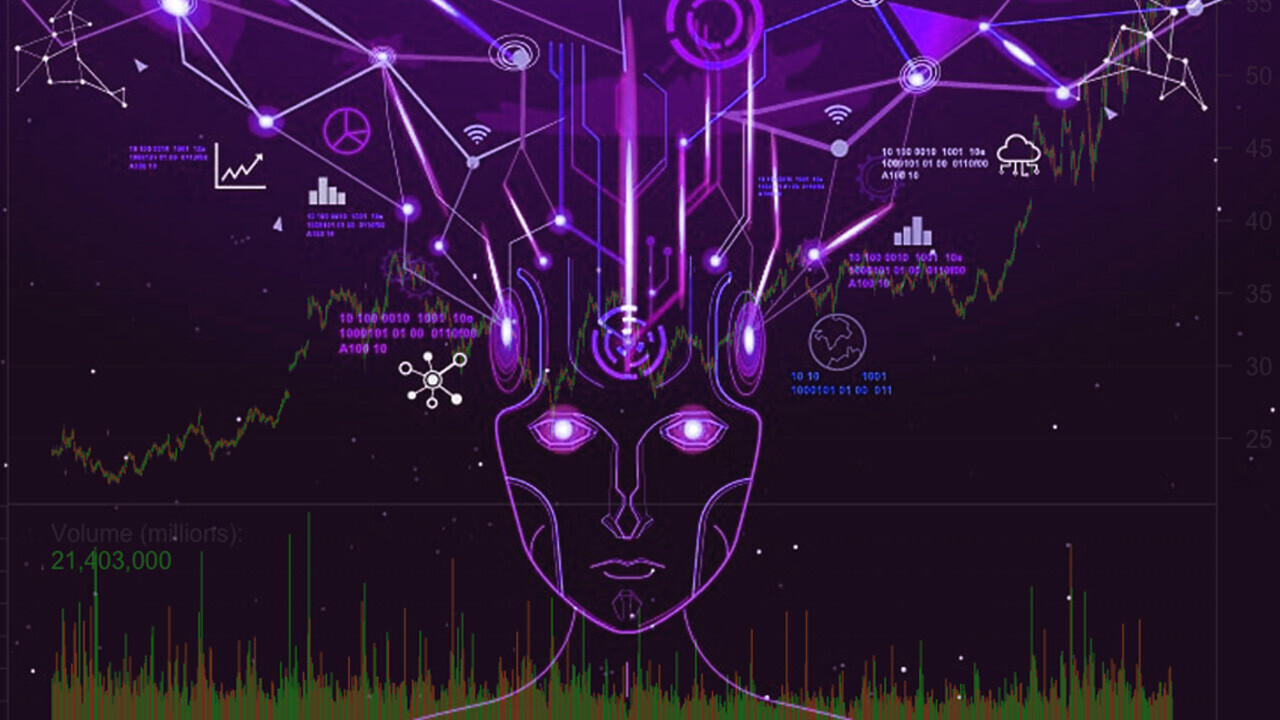
Natural language processing (NLP) is among the most exciting subsets of machine learning. It lets us talk to computers like they’re people and vice versa. Siri, Google Translate, and the helpful chat bot on your bank’s website are all powered by this kind of AI — but not all NLP systems are created equal.
In today’s AI landscape, smaller, targeted models trained on essential data are often better for business endeavors. However, there are massive NLP systems capable of incredible feats of communication. Called ‘large language models‘ (LLMs), these are capable of answering plain language queries, and generating novel text. Unfortunately, they’re mostly novelty acts unsuited for the kind of specialty work most professional organizations need from AI systems.
OpenAI’s GPT-3, one of the most popular LLMs, is a mighty feat of engineering. But it’s also prone to outputting text that’s subjective, inaccurate, or nonsensical. This makes these huge, popular models unfit for industries where accuracy is important.
A lucrative outlook
While there’s no such thing as a sure bet in the world of STEM, the forecast for NLP technologies in Europe is bright and sunny for the foreseeable future. The global market for NLP is estimated at about $13.5 billion today, but experts believe the market in Europe alone will swell to more than $21 billion by 2030.
This indicates a wide-open market for new startups to form alongside established industry actors, such as Dataiku and Arria NLG. The former, Dataiku, was initially formed in Paris, but managed to perform extremely well on the global funding stage and now has offices around the world. And the latter company, Arria NLG, is essentially a University of Aberdeen spinout that’s expanded well beyond its Scottish origins. Both companies have reached massive success on the back of their natural language processing solutions by focusing on data-centric solutions that produce verifiable, accurate, results for enterprise, pharma, and government services.
One reason for the massive success of these particular outlets is that it’s extremely difficult to train and build AI models that are trustworthy. An LLM trained on a massive dataset, for example, will tend to output ‘fake news’ in the form of random statements. This is useful when you’re looking for writing ideas or inspiration, but it’s entirely untenable when accuracy and factual outputs are important.
I spoke with Emmanuel Walckenaer, the CEO of one such company, Yseop. His Paris-based outfit is an AI startup that specializes in using NLP for natural language generation (NLG) in standardized industries such as pharma and finance. According to him, when it comes to building AI for these domains, there’s no margin for error. “It has to be perfect,” he told TNW.
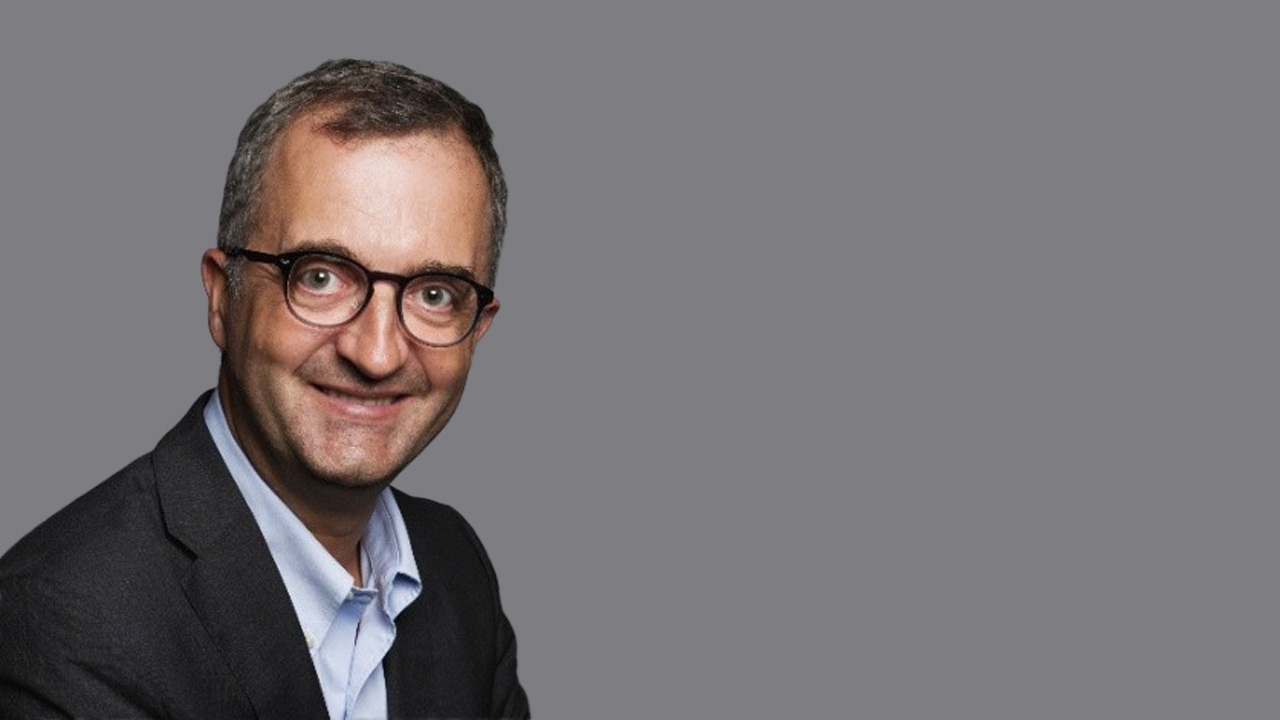
The problem with LLMs
You’d be hard-pressed to find a more popular topic among AI journalists in 2022 than LLMs such as GPT-3 and Google’s LaMBDA. For the first time in history, pundits can “talk” to a machine and that makes for fun, compelling articles. Not to mention the fact these models have gotten so good at imitating humans that some experts even think they’re becoming sentient.
While these systems are impressive, as mentioned above, they are usually completely untrustworthy. They’re brittle, unreliable, and prone to making things up. In layperson’s terms: they’re dumb liars. This is because of the way they are trained.
LLMs are amazing marriages of mathematics and linguistics. But, at their most basic, they’re beholden to the data they’re trained on. You can’t expect to train an AI on, for example, a corpus of Reddit posts, and not expect it to have some factual inconsistencies. As the old saying goes, you get out what you put in.
If, for example, you trained a LLM on a dataset full of cooking recipes, you could then develop a system capable of generating new recipes on demand. You might ask it to generate a novel recipe for something that isn’t in its database — such as, perhaps, a gummy bear curry.
Just like a human chef would have to tap into their cooking background in order to figure out how to integrate gummy bears into something resembling a curry dish, the AI would attempt to throw together a new recipe based on the ones it had been trained on. If it had been trained on a database of curry recipes, there’s a reasonable chance it’d output something at least close to what a human might come up with given the same task.
However, if the team training the AI used a giant dataset full of billions or trillions of internet files that have nothing to do with curry, there’s no telling what the machine might spit out. It might give you a great recipe, it might output a random diatribe on NBA superstar Stephen Curry.
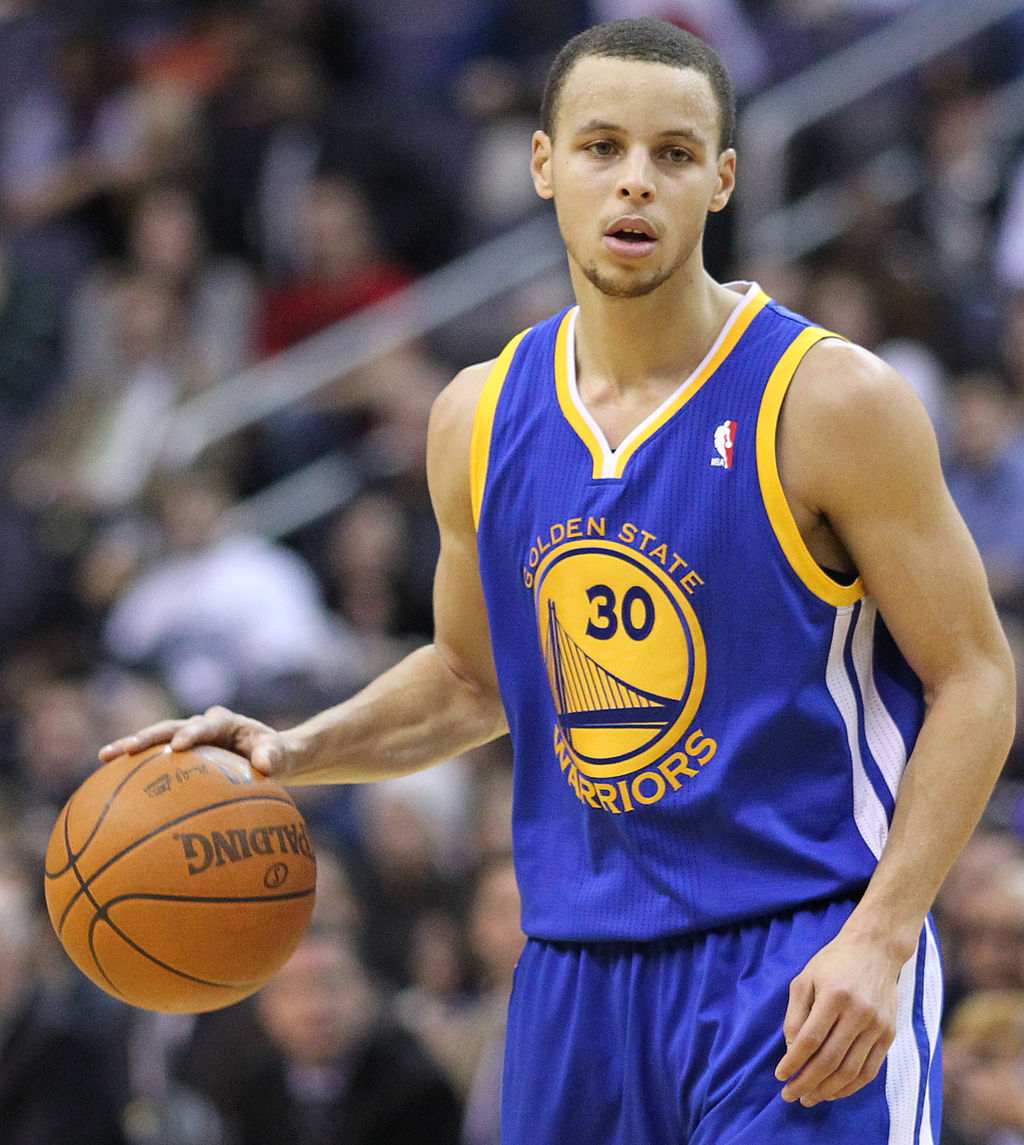
That’s sort of the fun part about working with huge LLMs, you never quite know what you’ll get when you query them. However, there’s no room for that kind of uncertainty in medical, financial, or business intelligence reports.
Reigning in human knowledge for machine use
The companies developing AI solutions for standardized industries don’t have the luxury of brute-force training giant models on the biggest databases around just to see what they’re capable of. The output from their systems is typically submitted for review by governing authorities such as the USFDA and global financial regulators. For this reason, these organizations have to be very careful about what kind of data they train their models on.
Walckenaer told me that Yseop’s first priority is ensuring the data they use to train their systems is both accurate and ethically sourced. This means using only the applicable data and ensuring that no human’s privacy is compromised by anonymizing it to remove any personally identifiable information.
Next, the company has to ensure its machine learning systems are free of bias, omission, and hallucination. Yes, you read that right: blackbox AI systems have a tendency to hallucinate, and that’s a huge problem if you’re trying to output information that’s 100% accurate.
To overcome the problem of hallucination, Yseop relies on having humans in the loop at every stage. The company’s algorithms and neural networks are co-developed by math wizards, linguistics experts, and AI developers. Their databases consist of data sourced directly from the researchers and businesses being served by the product. And the majority of their offerings are conducted via SaaS and designed to “augment” human professionals — as opposed to replacing them.
With humans involved at every stage, there are checks in place to ensure the AI doesn’t take the data it’s been given and “hallucinate” new, made-up information. This, for example, keeps the system from using real patient data as a template for outputting fake data about patients that don’t exist.
The next problem devs need to overcome with language processing is omission. This happens when an AI model skips over pertinent or essential parts of its database when it outputs information.
Massive LLMs such as GPT-3 don’t really suffer from the omission problem — you never know what to expect from these “anything goes” systems anyway. But targeted models that are designed to help professionals and businesses sort through finite datasets are only useful if they can be “containerized” in such a way as to surface all of the relevant information.
The last major hurdle that huge LLMs usually fail to pass is bias. One of the most common forms of bias is technical. This occurs when systems are designed in such a way that the outputs they produce don’t follow the scientific method.
A prime example of technical bias would be teaching a machine to “predict” a person’s sexuality. Since there’s no scientific basis for this kind of AI (see our article on why supposed “gaydars” are nothing but hogwash and snake oil), they’re only able to produce made-up outputs by employing pure technical bias.
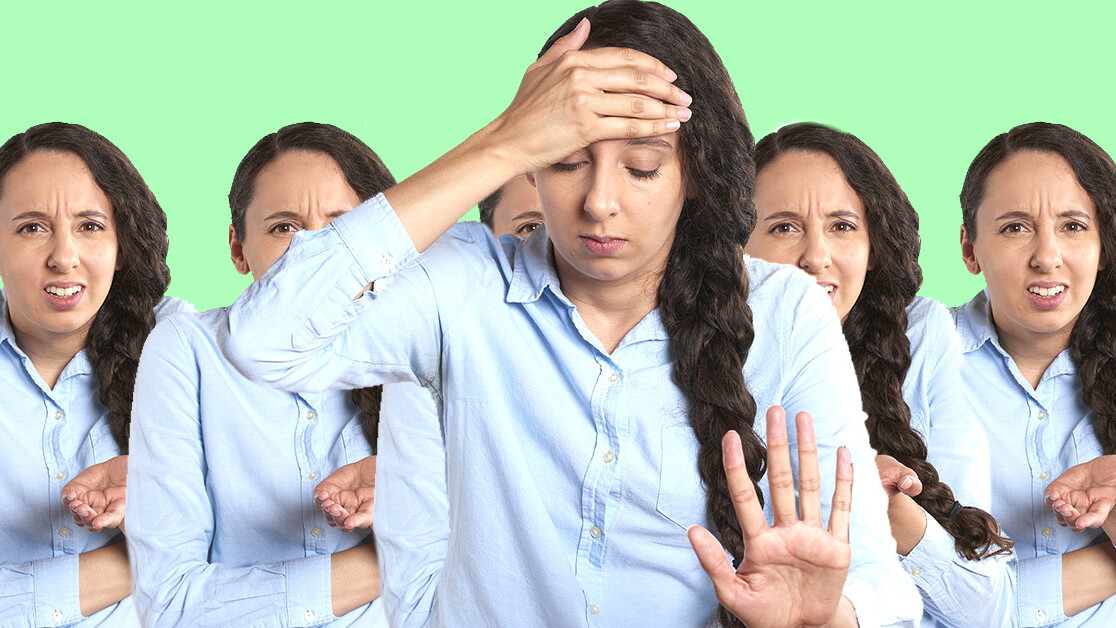
Other common forms of bias that can creep into NLP and NLG models include human bias — this happens when humans improperly label data due to cultural or intentional misinterpretation — and institutional bias.
The last one can be a huge problem for organizations that rely on accurate data and outputs to make important decisions. In standardized industries such as pharma and finance, this kind of bias can produce poor outcomes for patients and contribute to financial ruin. Suffice to say that bias is among the biggest problems in AI, and LLMs such as GPT-3 are, essentially, as biased as the databases they’re trained on.
Though it can be difficult to eliminate bias outright, it can be mitigated by using only the highest quality, hand-checked data, and ensuring that the system’s “parameters” — essentially, the virtual dials and knobs that allow developers to fine-tune an AI’s outputs — are properly adjusted.
GPT-3 and similar models are capable of mind-blowing feats of prose and, occasionally, they even fool some experts. But they’re entirely unsuited for standardized industries where accuracy and accountability are paramount.
Why use AI at all?
It can start to seem like a bad idea to employ LLMs or NLP/NLG at all when the stakes are high. In the pharmaceutical industry, for example, bias or omission could have a massive impact on the accuracy of clinical reports. And who wants to trust a machine that hallucinates with their financial future?
Luckily for all of us, companies such as Yseop don’t use open-ended datasets full of unchecked information. Sure, you’re unlikely to get Yseop’s pharma models to write a song or produce a decent curry recipe (with their current datasets), but, because the data and parameters governing their outputs are used with careful scrutiny, they can be trusted for the tasks they’re built for.
But it still begs the question, why use AI at all? We’ve gotten by this far with non-automated software solutions.
Walckenaer told me there may soon be no other choice. According to him, the human workforce can’t keep up — at least in the pharmaceutical industry.
“The need for medical writers is going to triple in the next ten years” says Walckenaer, who also added that Yseop’s systems can provide a 50% efficiency gain for applicable industries. That’s a game-changer. And there’s even good news for those who fear being displaced by machines. He assured us that Yseop’s systems were meant to augment skilled human labor, not replace people.
In other standardized industries, such as finance or in the domain of business intelligence, NLP and NLG can help minimize or even eliminate human error. That might not be as exciting as using a LLM capable of pretending to chat with you as a famous historical figure or generating fake news at the push of a button, but it currently saves thousands of businesses around the world time and money.
Get the TNW newsletter
Get the most important tech news in your inbox each week.